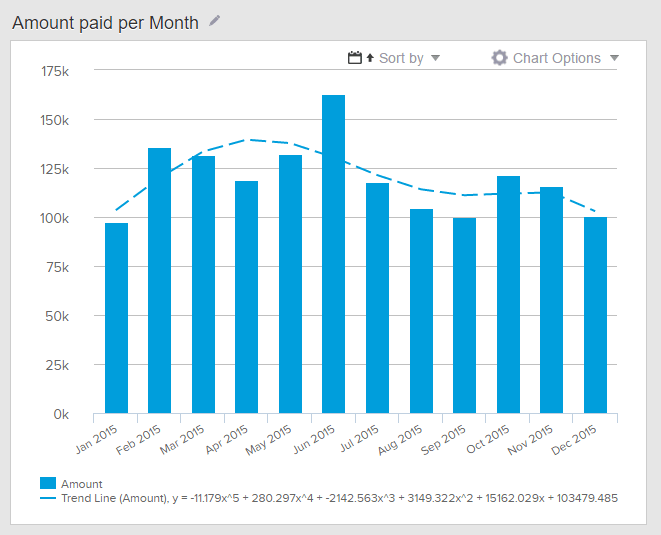
- R Losses Regression Analysis
- Loess Regression R Squared
- R Losses Regression Calculator
- R Loess Regression
LiblineaR can produce 10 types of (generalized) linear models, by combining several types of loss functions and regularization schemes. The regularization can be L1 or L2, and the losses can be the regular L2-loss for SVM (hinge loss), L1-loss for SVM, or the logistic loss for logistic regression. The default value for type is 0. See details below. ) from the gaze of R's formula parsing code. It allows the standard R operators to work as they would if you used them outside of a formula, rather than being treated as special formula operators. For example: y x + x^2 would, to R, mean 'give me: x = the main effect of x, and; x^2 = the main effect and the second order interaction of x'.
- R Tutorial
- R Data Interfaces
- R Charts & Graphs
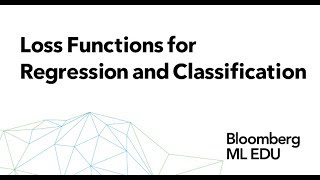
- R Statistics Examples
- R Useful Resources
- Selected Reading
Regression analysis is a very widely used statistical tool to establish a relationship model between two variables. One of these variable is called predictor variable whose value is gathered through experiments. The other variable is called response variable whose value is derived from the predictor variable.
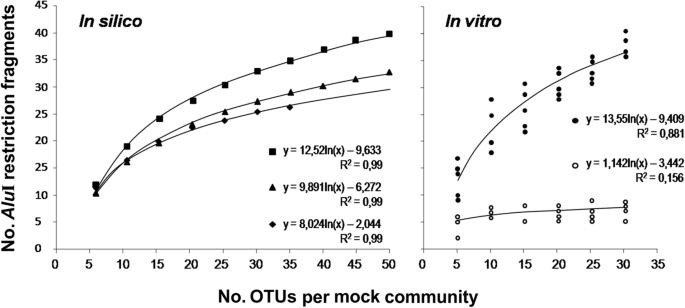
In Linear Regression these two variables are related through an equation, where exponent (power) of both these variables is 1. Mathematically a linear relationship represents a straight line when plotted as a graph. A non-linear relationship where the exponent of any variable is not equal to 1 creates a curve.
The general mathematical equation for a linear regression is −
Following is the description of the parameters used −
y is the response variable.
x is the predictor variable.
a and b are constants which are called the coefficients.
Steps to Establish a Regression
A simple example of regression is predicting weight of a person when his height is known. To do this we need to have the relationship between height and weight of a person.
The steps to create the relationship is −
Carry out the experiment of gathering a sample of observed values of height and corresponding weight.
Create a relationship model using the lm() functions in R.
Find the coefficients from the model created and create the mathematical equation using these
Download Slots Era and join the lucky play today, winner:-) The Slots Era Team. Install one of the best casino games now for FREE and get a huge welcome Bonus of 12,000,000 Coins. New Era of Las Vegas Slot Games has come! Slots era hack apk download pc windows.
Get a summary of the relationship model to know the average error in prediction. Also called residuals.
To predict the weight of new persons, use the predict() function in R. Slots era freebies online.
Input Data

Below is the sample data representing the observations −
lm() Function
This function creates the relationship model between the predictor and the response variable.
Syntax
The basic syntax for lm() function in linear regression is −
Following is the description of the parameters used −
formula is a symbol presenting the relation between x and y.
data is the vector on which the formula will be applied.
Create Relationship Model & get the Coefficients
When we execute the above code, it produces the following result −
Get the Summary of the Relationship
When we execute the above code, it produces the following result −
predict() Function
Syntax
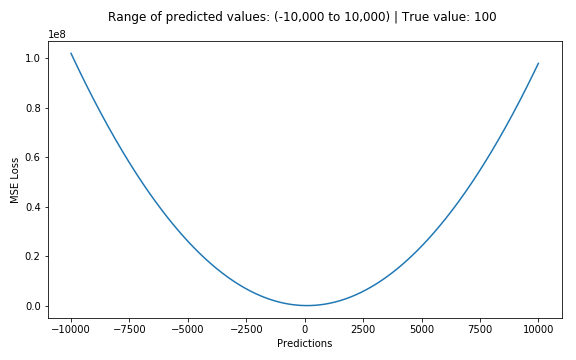
- R Losses Regression Analysis
- Loess Regression R Squared
- R Losses Regression Calculator
- R Loess Regression
LiblineaR can produce 10 types of (generalized) linear models, by combining several types of loss functions and regularization schemes. The regularization can be L1 or L2, and the losses can be the regular L2-loss for SVM (hinge loss), L1-loss for SVM, or the logistic loss for logistic regression. The default value for type is 0. See details below. ) from the gaze of R's formula parsing code. It allows the standard R operators to work as they would if you used them outside of a formula, rather than being treated as special formula operators. For example: y x + x^2 would, to R, mean 'give me: x = the main effect of x, and; x^2 = the main effect and the second order interaction of x'.
- R Tutorial
- R Data Interfaces
- R Charts & Graphs
- R Statistics Examples
- R Useful Resources
- Selected Reading
Regression analysis is a very widely used statistical tool to establish a relationship model between two variables. One of these variable is called predictor variable whose value is gathered through experiments. The other variable is called response variable whose value is derived from the predictor variable.
In Linear Regression these two variables are related through an equation, where exponent (power) of both these variables is 1. Mathematically a linear relationship represents a straight line when plotted as a graph. A non-linear relationship where the exponent of any variable is not equal to 1 creates a curve.
The general mathematical equation for a linear regression is −
Following is the description of the parameters used −
y is the response variable.
x is the predictor variable.
a and b are constants which are called the coefficients.
Steps to Establish a Regression
A simple example of regression is predicting weight of a person when his height is known. To do this we need to have the relationship between height and weight of a person.
The steps to create the relationship is −
Carry out the experiment of gathering a sample of observed values of height and corresponding weight.
Create a relationship model using the lm() functions in R.
Find the coefficients from the model created and create the mathematical equation using these
Download Slots Era and join the lucky play today, winner:-) The Slots Era Team. Install one of the best casino games now for FREE and get a huge welcome Bonus of 12,000,000 Coins. New Era of Las Vegas Slot Games has come! Slots era hack apk download pc windows.
Get a summary of the relationship model to know the average error in prediction. Also called residuals.
To predict the weight of new persons, use the predict() function in R. Slots era freebies online.
Input Data
Below is the sample data representing the observations −
lm() Function
This function creates the relationship model between the predictor and the response variable.
Syntax
The basic syntax for lm() function in linear regression is −
Following is the description of the parameters used −
formula is a symbol presenting the relation between x and y.
data is the vector on which the formula will be applied.
Create Relationship Model & get the Coefficients
When we execute the above code, it produces the following result −
Get the Summary of the Relationship
When we execute the above code, it produces the following result −
predict() Function
Syntax
The basic syntax for predict() in linear regression is −
Following is the description of the parameters used −
R Losses Regression Analysis
object is the formula which is already created using the lm() function.
newdata is the vector containing the new value for predictor variable.
Predict the weight of new persons
Loess Regression R Squared
When we execute the above code, it produces the following result −
R Losses Regression Calculator
Visualize the Regression Graphically
R Loess Regression
When we execute the above code, it produces the following result −